This course will introduce the learner to applied machine learning, focusing more on the techniques and methods than on the statistics behind these methods. The course will start with a discussion of how machine learning is different than descriptive statistics, and introduce the scikit learn toolkit through a tutorial. The issue of dimensionality of data will be discussed, and the task of clustering data, as well as evaluating those clusters, will be tackled. Supervised approaches for creating predictive models will be described, and learners will be able to apply the scikit learn predictive modelling methods while understanding process issues related to data generalizability (e.g. cross validation, overfitting). The course will end with a look at more advanced techniques, such as building ensembles, and practical limitations of predictive models. By the end of this course, students will be able to identify the difference between a supervised (classification) and unsupervised (clustering) technique, identify which technique they need to apply for a particular dataset and need, engineer features to meet that need, and write python code to carry out an analysis.

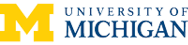
Applied Machine Learning in Python
This course is part of Applied Data Science with Python Specialization
Taught in English
Some content may not be translated

Instructor: Kevyn Collins-Thompson
294,116 already enrolled
Included with
Course
(8,466 reviews)
92%
What you'll learn
Describe how machine learning is different than descriptive statistics
Create and evaluate data clusters
Explain different approaches for creating predictive models
Build features that meet analysis needs
Details to know

Add to your LinkedIn profile
4 quizzes
Course
(8,466 reviews)
92%
See how employees at top companies are mastering in-demand skills
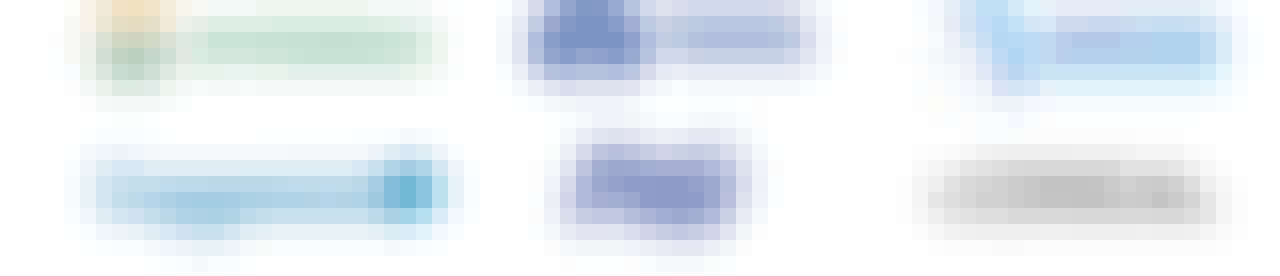
Build your subject-matter expertise
- Learn new concepts from industry experts
- Gain a foundational understanding of a subject or tool
- Develop job-relevant skills with hands-on projects
- Earn a shareable career certificate
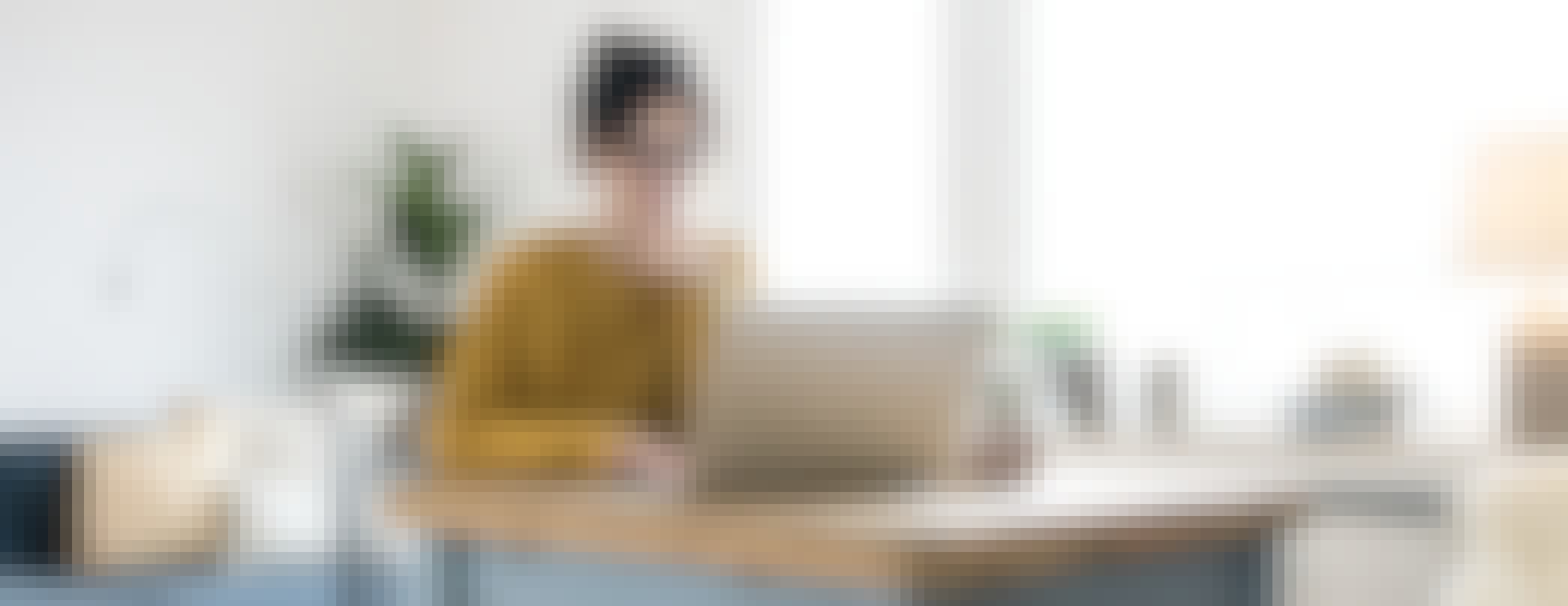
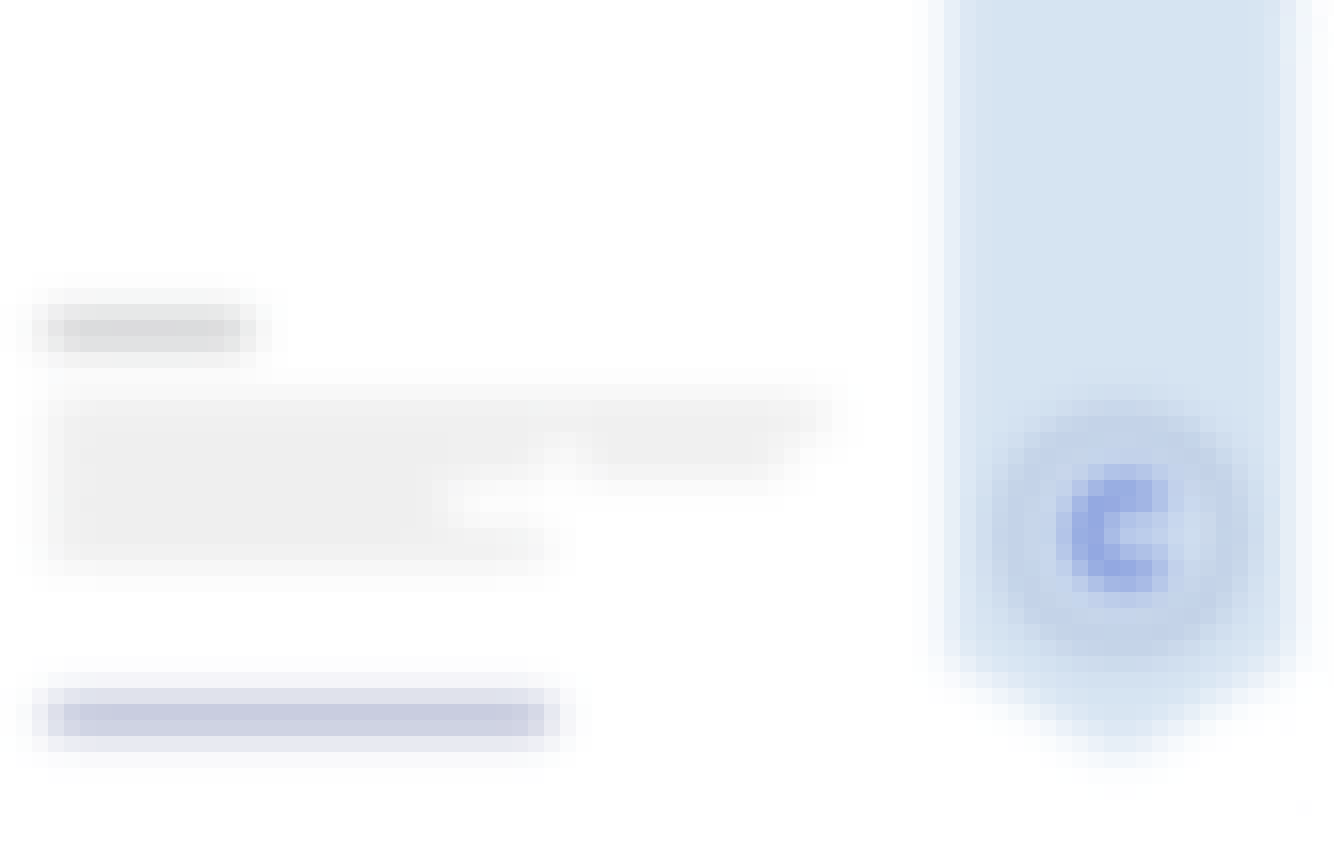
Earn a career certificate
Add this credential to your LinkedIn profile, resume, or CV
Share it on social media and in your performance review
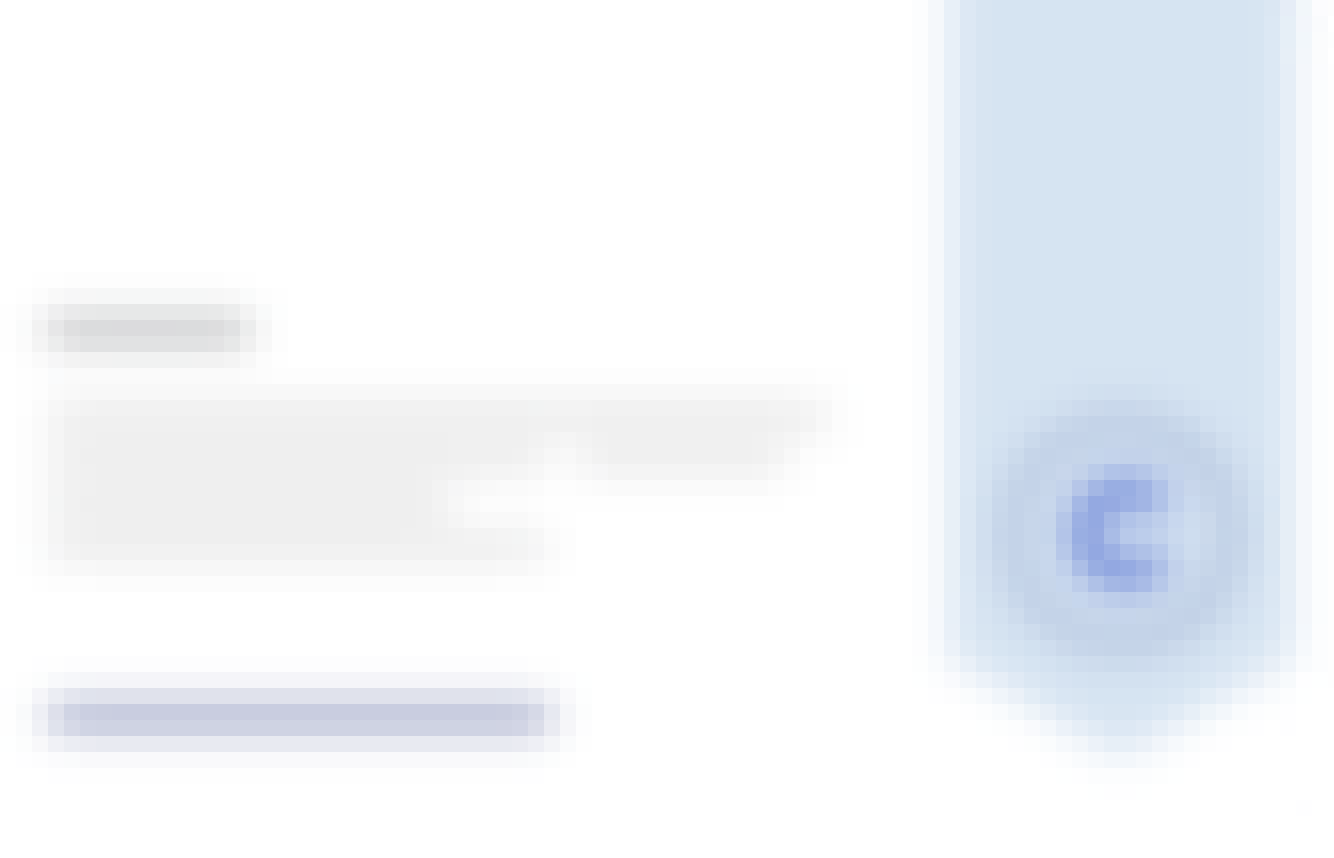
There are 4 modules in this course
This module introduces basic machine learning concepts, tasks, and workflow using an example classification problem based on the K-nearest neighbors method, and implemented using the scikit-learn library.
What's included
7 videos4 readings1 quiz1 programming assignment1 ungraded lab
This module delves into a wider variety of supervised learning methods for both classification and regression, learning about the connection between model complexity and generalization performance, the importance of proper feature scaling, and how to control model complexity by applying techniques like regularization to avoid overfitting. In addition to k-nearest neighbors, this week covers linear regression (least-squares, ridge, lasso, and polynomial regression), logistic regression, support vector machines, the use of cross-validation for model evaluation, and decision trees.
What's included
13 videos2 readings1 quiz1 programming assignment2 ungraded labs
This module covers evaluation and model selection methods that you can use to help understand and optimize the performance of your machine learning models.
What's included
8 videos2 readings1 quiz1 programming assignment1 ungraded lab
This module covers more advanced supervised learning methods that include ensembles of trees (random forests, gradient boosted trees), and neural networks (with an optional summary on deep learning). You will also learn about the critical problem of data leakage in machine learning and how to detect and avoid it.
What's included
10 videos13 readings1 quiz1 programming assignment2 ungraded labs
Instructor

Offered by
Recommended if you're interested in Data Analysis
University of Michigan
University of Michigan
University of Michigan
University of Michigan
Prepare for a degree
Taking this course by University of Michigan may provide you with a preview of the topics, materials and instructors in a related degree program which can help you decide if the topic or university is right for you.
Why people choose Coursera for their career




Learner reviews
Showing 3 of 8466
8,466 reviews
- 5 stars
71.61%
- 4 stars
21.03%
- 3 stars
4.92%
- 2 stars
1.16%
- 1 star
1.26%
New to Data Analysis? Start here.

Open new doors with Coursera Plus
Unlimited access to 7,000+ world-class courses, hands-on projects, and job-ready certificate programs - all included in your subscription
Advance your career with an online degree
Earn a degree from world-class universities - 100% online
Join over 3,400 global companies that choose Coursera for Business
Upskill your employees to excel in the digital economy
Frequently asked questions
Access to lectures and assignments depends on your type of enrollment. If you take a course in audit mode, you will be able to see most course materials for free. To access graded assignments and to earn a Certificate, you will need to purchase the Certificate experience, during or after your audit. If you don't see the audit option:
The course may not offer an audit option. You can try a Free Trial instead, or apply for Financial Aid.
The course may offer 'Full Course, No Certificate' instead. This option lets you see all course materials, submit required assessments, and get a final grade. This also means that you will not be able to purchase a Certificate experience.
When you enroll in the course, you get access to all of the courses in the Specialization, and you earn a certificate when you complete the work. Your electronic Certificate will be added to your Accomplishments page - from there, you can print your Certificate or add it to your LinkedIn profile. If you only want to read and view the course content, you can audit the course for free.
If you subscribed, you get a 7-day free trial during which you can cancel at no penalty. After that, we don’t give refunds, but you can cancel your subscription at any time. See our full refund policy.